Autore:
D. Cucina, A. di Salvatore, M. K. Protopapas
Abstract:
Meta-heuristic methods to detect multiple additive outliers in multivariate time series are proposed. The implemented algorithms are: simulated annealing, threshold accepting and two different versions of genetic algorithms. In contrast with many of the existing methods, they do not require to specify a vector ARMA model for the data and are able to detect any number of potential outliers simultaneously reducing possible masking and swamping effects. A generalized AIC-like criterion has been used as objective function where the penalty constant has been suggested by both a simulation study and a theoretical approximation. The comparison and the performance of the proposed methods are illustrated by simulation studies and real data analysis. Simulation results show that the proposed approaches are able to handle patches of additive outliers.
Parole Chiave:
Genetic algorithms, Simulated annealing, Threshold accepting
Tipo di pubblicazione:
Rapporto Tecnico
Codice Pubblicazione:
4
Allegato Pubblicazione:
Contatto:
ISSN:
2279-798X
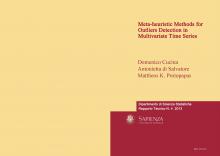